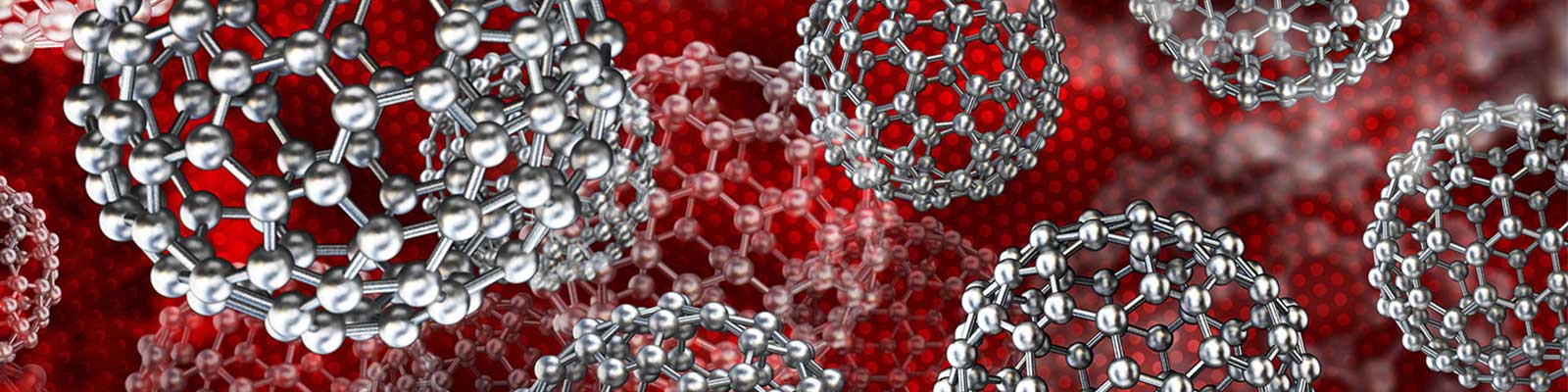
Resources
Below is a broad but not exhaustive overview of current and recent resources available from the Medical Engineering Research team.
Objectively recognizing eating behaviour and associated intake
Automatic detection of intake gestures is a key element of automatic dietary monitoring. Several types of sensors, including inertial measurement units (IMU) and video cameras have been used for this purpose.
The common machine learning approaches make use of the labelled sensor data to automatically learn how to make detections.
One characteristic, especially for deep learning models, is the need for large datasets. To meet this need, we collected the Objectively Recognizing Eating Behaviour and Associated Intake (OREBA) dataset.
The OREBA dataset aims to provide a comprehensive multi-sensor recording of communal intake occasions for researchers interested in automatic detection of intake gestures and other behaviours associated with intake.
Two scenarios are included, with 100 participants for an individual dish and 102 participants for a shared dish. Available sensor data consists of synchronised frontal video and IMU with accelerometer and gyroscope for both hands.
Get access
The OREBA dataset is available for research groups. Please download the agreement form below, complete it, and return it to OREBA@newcastle.edu.au. We will get back to you with a download link and password.
Download
OREBA Agreement Form
Publications
If you use the OREBA dataset, please consider citing our publications.
Bibtex Referencing Format:
APA Referencing Format:
Rouast, P. V., Heydarian, H., Adam, M. T. P., Rollo, M. (2020). OREBA: A dataset for objectively recognizing eating behaviour and associated intake. Working Paper, 1-5. https://arxiv.org/abs/2007.15831
Rouast, P.B., Adam, M. T. P. (2020). Learning deep representations for video-based intake gesture detection. IEEE Journal of Biomedical and Health Informatics, 24(6), 1727–1737. https://www.doi.org/10.1109/JBHI.2019.2942845
The University of Newcastle acknowledges the traditional custodians of the lands within our footprint areas: Awabakal, Darkinjung, Biripai, Worimi, Wonnarua, and Eora Nations. We also pay respect to the wisdom of our Elders past and present.